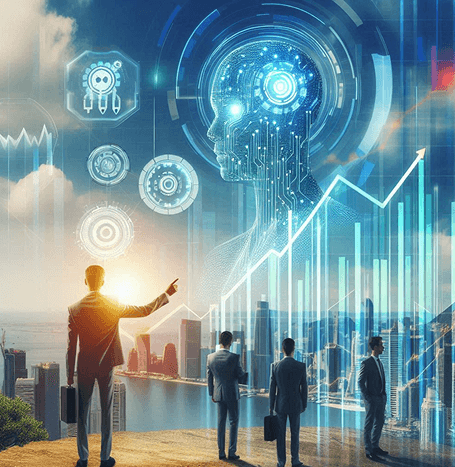
Unlocking new opportunities and business success through data-driven decision-making
In an era where technology and data are shaping the future of industries, businesses are increasingly turning to Artificial Intelligence (AI) to enhance decision-making. In today’s fast-paced digital world, making informed decisions is critical for businesses seeking to remain competitive and innovative. One of the most effective ways to do this is through data-driven decision-making. Data-Driven Decision Making (DDDM) is the process of making organizational decisions based on actual data rather than intuition, gut feelings, or personal experience. It involves collecting, analyzing, and interpreting data to guide strategic choices, improve business outcomes, and solve problems. This approach leverages data and analytics to guide strategic decisions, ensuring that choices are based on objective insights rather than gut feelings or assumptions. Data-driven decision-making refers to the process of collecting, analyzing, and using data to guide business decisions.
Why It Matters
By utilizing real-time data, businesses can better understand market trends, consumer behavior, and internal performance. Whether optimizing marketing strategies, improving product offerings, or streamlining operations, DDDM provides the foundation for making well-informed decisions that can lead to more predictable outcomes. Data-driven decision making is superior to other forms of decision making because it ensures that decisions are based on logic and reasoning as well as supported by numerical evidence. Without the use of data to inform decisions, decision makers run the risk of being subconsciously influenced by factors such as bias, external stimuli such as the opinions/demands of others, or acting on assumptions, gut-feeling or theory. There are numerous benefits to using data to influence the decision-making process.
- Improved Accuracy: Decisions based on data tend to be more objective and accurate than decisions based on gut feelings or personal experience. Relying on evidence based data, reduces the chance of human error, bias, or assumptions influencing decisions. Data presents objective facts that are analyzed to provide actionable insights
- Informed Strategy: With data insights, organizations can make strategic decisions that are aligned with market trends, customer behaviors, and operational efficiencies.
- Increased Efficiency: Data can reveal inefficiencies in processes, allowing companies to optimize operations, cut costs, and improve performance. With access to data, businesses can identify inefficiencies within processes, adjust strategies quickly, and allocate resources effectively. This leads to better operational efficiency and cost savings.
- Competitive Edge: Organizations that leverage data effectively often have a competitive advantage by anticipating market changes, improving customer satisfaction, and innovating faster. Companies that effectively use data to drive decisions often gain a significant competitive edge. They can identify opportunities faster, react to market trends with agility, and improve their offerings based on customer feedback.
- Reduced Risk: Data-driven insights can help businesses identify potential risks early and take preventive action to mitigate them. Data analysis enables companies to better anticipate risks and challenges. Historical trends and predictive analytics help businesses prepare for potential disruptions or market changes.
- Enhanced Customer Understanding: Data from customer interactions, feedback, and purchasing behaviors helps businesses personalize experiences and predict future needs, creating a more customer-centric approach.
Who Has Benefited from Data-Driven Decision Making (DDDM) Before?
Several organizations across various industries have successfully leveraged data-driven decision-making (DDDM) to enhance their operations and achieve significant growth. Here are notable examples:
Amazon (Retail Industry)
- How DDDM Helped: Amazon revolutionized the retail industry by using customer behavior data to optimize product recommendations, manage inventory, and refine pricing strategies. Its recommendation algorithm, which suggests products based on users' past behavior, increased sales and customer satisfaction.
- Impact: This data-driven approach has significantly boosted sales, improved customer retention, and enhanced operational efficiency, positioning Amazon as a leader in e-commerce.
Johns Hopkins Medicine (Healthcare)
- How DDDM Helped: Johns Hopkins uses data to improve patient care by identifying trends in medical conditions and treatments. By analyzing patient data, they were able to reduce surgical complications and improve patient outcomes.
- Impact: The healthcare system reduced complications by 18%, improved care quality, and minimized risks for patients.
Johns Hopkins Medicine (Healthcare)
A large U.S.-based construction company, leveraged predictive analytics to identify potential risks and delays in a complex high-rise project. By analyzing various data sources, including weather patterns, labor productivity, and material availability, the company was able to proactively address issues before they escalated.
Through the use of predictive analytics, Mortenson was able to:
- Complete the 365 Nicollet project ahead of schedule
- Reduce overall project costs by minimizing delays and optimizing resource allocation
- Improve safety performance by anticipating and mitigating potential hazards
How Data-Driven Decision Making is Done
Data-Driven Decision Making (DDDM) is done through a systematic process of collecting, analyzing, and leveraging data to inform and optimize decision-making. Here's how it's done in detail:
Data Collection
Identify Relevant Data Sources: The first step is to determine what types of data are most relevant to the business objectives or the specific problem being addressed. These could include customer behavior, sales trends, website analytics, social media engagement, or operational metrics. Gather Data from Multiple Channels: Collect data from various sources such as:
- Internal systems (CRM, ERP, transaction databases)
- Customer interactions (feedback, surveys, support tickets)
- Web analytics tools (Google Analytics, Mixpanel)
- External sources (market research reports, social media, public datasets)
- Internet of Things (IoT) devices, sensors, and logs in manufacturing or tech.
Data Cleaning and Preparation
Raw data often contains errors, duplicates, or missing values. Cleaning the data ensures that the analysis will be based on accurate, reliable information. This involves: Removing duplicate or irrelevant data. Filling in missing values or removing incomplete records, correcting inconsistencies in formatting or data entry. Through structuring the data, we can systematically organize the cleaned data into a format suitable for analysis, often in tables or databases, making it easy to query and manipulate. Data-driven decision-making relies heavily on the processes of data cleaning and preparation. These foundational steps are essential for ensuring that the data used for analysis is accurate, complete, and relevant.
The significance of data cleaning cannot be overstated; it addresses various issues such as inconsistencies, errors, missing values, and outliers that can skew analysis. By investing time in cleaning data, organizations can enhance the quality and reliability of their insights, leading to more informed decision-making. Once data is gathered, the next phase involves discovery and profiling, where analysts assess the datasets to understand their structure and identify any quality issues.
Data Analysis
Before diving deep, it's important to perform an initial exploration of the data to understand its structure, distribution, and key characteristics. This can involve: Descriptive statistics (mean, median, mode, variance) and Visualizations (charts, graphs) to identify trends, correlations, and outliers.
Statistical Analysis: Depending on the type of data and the questions you're trying to answer, different statistical techniques are used, such as: Descriptive Analysis: Summarizes the current state of the data (e.g., average sales, customer demographics) another is Diagnostic Analysis: Looks at past performance to determine why something happened (e.g., why sales dropped last quarter).Predictive Analysis: Uses historical data to make predictions about future outcomes (e.g., forecasting next month’s sales based on past trends). Prescriptive Analysis: Suggests actions based on the analysis (e.g., recommending pricing strategies to increase revenue).
Data Visualization
Create Visual Dashboards: Using visualization tools (Tableau, Power BI, Google Data Studio), create dashboards that represent the insights from the analysis. Data visualizations such as bar charts, line graphs, heat maps, and pie charts help stakeholders quickly grasp complex data. It is through visualization that a lot of ideas can be fully materialized. Visualizations emphasize the important findings or trends, making it easier to communicate insights to decision-makers.
Develop Hypotheses and Test
Formulate Hypotheses: Based on the analysis, develop hypotheses about potential actions or strategies that could be taken (e.g., “Improving our website’s load time will increase customer conversion rates”). Experimentation (A/B Testing): Test these hypotheses through controlled experiments. For example, run an A/B test to compare the impact of different marketing strategies, pricing models, or product features.
Analyze Test Results: Use the data from these tests to understand which approach performs best and make decisions based on the results.
Make Data-Driven Decisions
Align Decisions with Insights: After analyzing and interpreting the data, use the insights to inform decisions. This could involve:
- Optimizing marketing campaigns based on customer preferences
- Adjusting pricing models to maximize profitability
- Streamlining operations by eliminating inefficiencies revealed in the data
After analyzing the data, ensure that key stakeholders (managers, employees, executives) are involved in the decision-making process and understand the data-driven insights.
Implement and Monitor
It is through strict and meticulous rules that a lot can be monitored. Implement the decisions made based on the data. Whether it's launching a new product, changing marketing strategies, or adjusting operations, data-driven decisions should be integrated into the business workflow.
Data analysis should be a continuous process. Use real-time or periodic monitoring to track the impact of the decision and ensure that it leads to the desired outcomes. Set KPIs (Key Performance Indicators) to measure the effectiveness of decisions and continuously collect new data to refine and adjust strategies.
Refinement and Iteration
After decisions have been implemented and executed, make sure to assess and measure their success by comparing the results against the initial goals or KPIs. If the data shows that the desired results weren’t achieved, refine the approach, make necessary adjustments, and test again. DDDM is iterative, requiring continuous improvement and adaptation as new data emerges.
How to Ensure Your Business Can Start Using Data to Drive Decision Making
Making informed decisions is more critical than ever in today's competitive business environment. Yet many organizations rely more on intuition than hard evidence. This is where the power of data-driven decision-making (DDDM) comes into play.
By harnessing the wealth of information, businesses can gain valuable insights, identify trends, and anticipate a customer's journey and needs. This article will explore DDDM's transformative potential and how it can strengthen your business operations, driving growth and success in today's competitive landscape. These are some of the factors and let's dive in:
Define Business Goals
Why It Matters: Start by identifying key business challenges or objectives that data can help solve. Without clear goals, data collection and analysis may be unfocused or irrelevant.
Foster a Data-Driven Culture
Why It Matters: The success of DDDM hinges on the mindset of your team. A data-driven culture promotes accountability, critical thinking, and evidence-based decisions. Make sure to train employees to understand and use data in their decision-making processes. Encourage managers to make decisions based on data rather than intuition. Create a culture where opinions are backed by data insights.
Create a Data-Driven Culture
Why It Matters: To make data-driven decisions a core part of your business, it needs to be ingrained in the company culture. Encourage teams to base their decisions on data-backed insights rather than assumptions or intuition. Set an example from leadership by using data in strategic discussions and planning. Reward data-driven decisions that positively impact business outcomes. And regularly discuss data performance and insights during team meetings to promote a data-centric approach.
Monitor and Adapt
Why It Matters: Data-driven decision-making is an ongoing process. You need to continuously monitor performance, track results, and adjust your strategy as new data becomes available. To make sure everything follows, implement real-time dashboards that provide a clear picture of your KPIs and performance metrics. Set up periodic reviews to assess the effectiveness of data-driven decisions and make sure to stay agile by using new data to iterate on strategies, improve decision-making, and adjust to changing market conditions.
Collaborate with Data Experts or Consultants
Why It Matters: If you’re new to data analytics or don’t have in-house expertise, partnering with data experts can speed up the transition to a data-driven approach.
Hire data scientists, data engineers, or data analysts to help you collect, clean, and analyze data. Alternatively, partner with a data consultancy to set up your infrastructure, train your team, or conduct specific data analysis projects and use their insights and expertise to build a long-term data strategy that aligns with your business objectives.
By following these steps, you'll set a strong foundation for becoming a data-driven business, enhancing your ability to make more informed, accurate, and impactful decisions. At DataBit, we always make sure that every client is unique and so are their needs. By leveraging AI, businesses can accelerate AI adoption, reduce risks, and unlock new growth opportunities through cutting-edge technology and expert guidance. We make sure to offer commitment and personalized service to achieve a desirable outcome. With us, we make sure that you’re not just another project — you’re a partner.